
Picture by upklyak on Freepik
Companies spend billions yearly on promoting to extend their product consciousness and curiosity amongst customers resulting in extra purchases. Focused ads or campaigns are launched to succeed in a broader viewers to be able to purchase new clients for his or her merchandise. Commercials are broadcasted by way of a number of media, it may be broadcasted on tv, radio, magazines, on-line, by way of social media, and even in shops to succeed in a large viewers. As a result of abundance of choices and the crucial to maximise return on funding (ROI), environment friendly allocation of promoting assets presents a major problem. That is the place media combine modeling turns into paramount for companies to optimize their commercial spending and maximize their RoI. By estimating how a lot cash needs to be spent on sure media channels to attain particular targets (comparable to elevated gross sales or model consciousness), media combine modeling is a useful gizmo for companies. This enables them to match numerous channels’ efficiency and determine the place there are alternatives for enchancment, and spend assets sensibly. Information-driven insights permit companies to fine-tune their advertising approaches, improve the worth of their spending, and speed up the method of reaching their enterprise aims. Media combine modeling is a statistical evaluation approach the place one analyzes historic commercial information together with spend, advert impressions or clicks delivered, product gross sales, new clients acquired, and so on. to be able to perceive the impression of various media channels on enterprise outcomes. This enables companies to carry out their commercial price range portfolio optimization and improve their RoI.
Along with the impression of media channels, media combine modeling additionally takes under consideration exterior components comparable to if the enterprise ran any promotions, holidays, or any particular occasion which could have impacted the gross sales. That is executed to be able to keep away from any type of overestimation of the impression of the media channels’ efficiency.
However one of the crucial important components that companies want to deal with and incorporate into their media combine fashions is Seasonality.
In easy phrases, we are able to outline seasonality as a attribute of time collection information through which we are able to observe predictable and common conduct that will get repeated yearly. Thus, we are able to say that any behavioral fluctuation that’s predictable and recurs yearly is a seasonal conduct.
However, how does seasonality differ from cyclical impact? Cyclical results are those that may span over various time durations. They could last more or shorter than one yr comparable to boosted gross sales of water filtration gadgets in a area as a consequence of some fault which may have occurred within the water pipeline. This type of impact isn’t common or predictable and may not repeat yearly. Whereas, a lift in gross sales of flu drugs as a consequence of flu season could be characterised as seasonal because it repeats yearly from December to February and could be predicted.
However why is seasonality vital for media promoting? Seasonality primarily impacts media combine modeling in two methods. Firstly, a change in media consumption patterns, and secondly a shift in promoting effectiveness is noticed. As we mentioned above how media combine modeling may help entrepreneurs perceive the impression of varied media channels on their gross sales or different key efficiency indicators comparable to new buyer acquisitions. The incorporation of seasonality into these fashions allows advertisers to extra precisely mirror the shifts in promoting efficiency that happen all year long. As an illustration, throughout the vacation seasons, numerous media channels could observe elevated viewership or increased engagement, which makes them extra profitable in reaching their respective goal audiences. Advertisers are in a position to maximize the effectiveness of their media allocation plans once they acknowledge and bear in mind seasonality. They can modify their promoting budgets, campaigns, and channel choice to be able to align themselves with seasonal tendencies and the demand of customers. This ensures that advertising efforts are concentrated throughout instances when they’re probably to generate most impression, therefore optimizing the return on promoting investments.
We are able to incorporate seasonality into media combine fashions through the use of seasonal components or dummy variables to symbolize particular seasonal occasions comparable to holidays. These components seize the affect of various time durations on media response and assist modify the mannequin’s predictions accordingly. These variables seize the impression of various time durations on media response and assist within the mannequin’s prediction.
In Python, now we have a Statsmodel library often known as seasonal_decompose, that may assist generate seasonality variables for us. The library splits a time collection into three elements specifically, pattern, seasonality, and the residuals. Seasonality could be represented by two sorts of fashions, both additive or multiplicative.
For simplicity, allow us to assume we’re coping with an additive mannequin. An additive mannequin can be utilized when the variance of the time collection does not change over totally different values of the time collection. Mathematically we are able to symbolize an additive mannequin as follows the place the person elements of pattern, seasonality, and residual are added collectively.

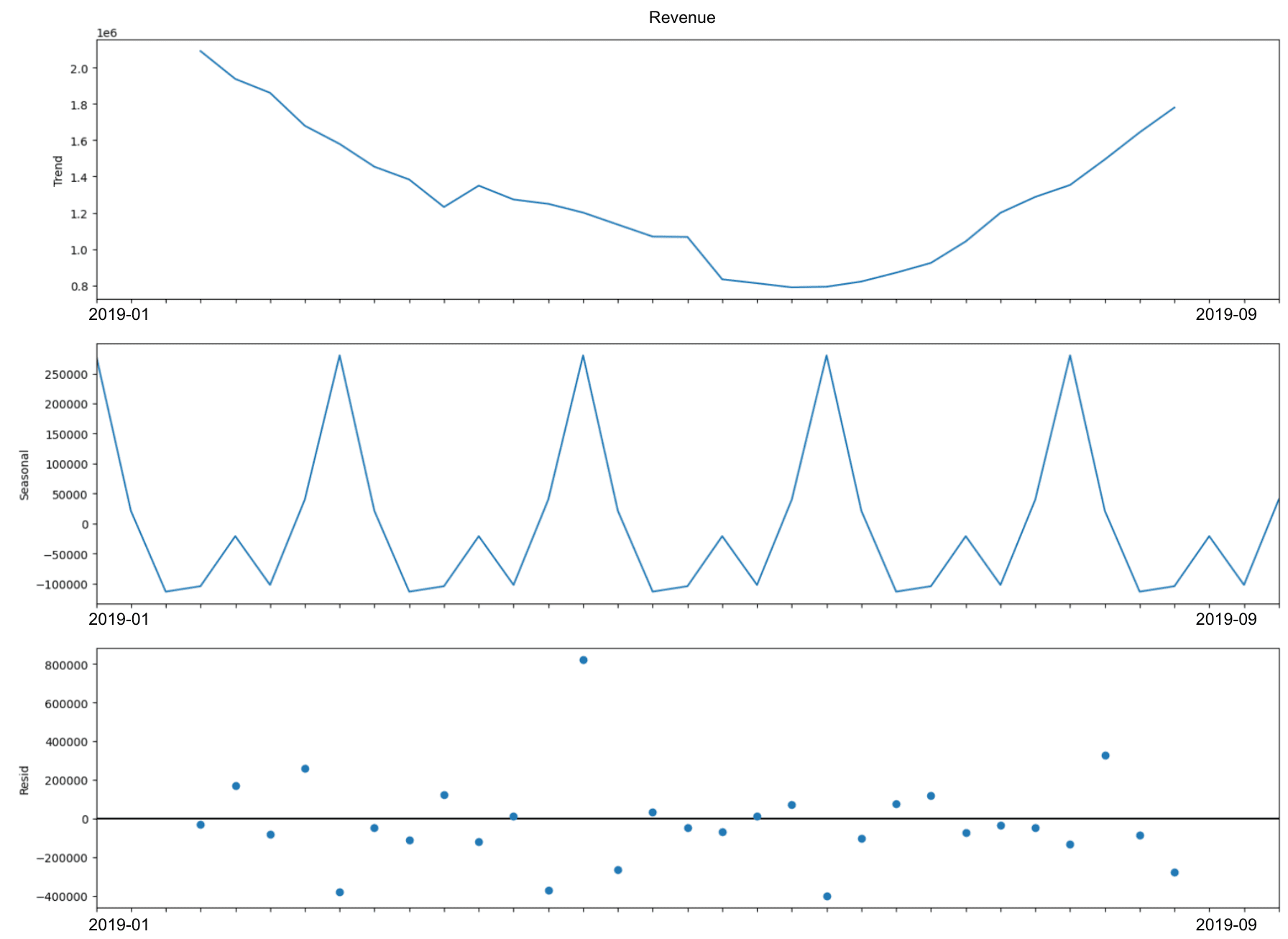
Determine 1: Seasonality decomposition of income over 8 months
Pattern Issue
The pattern element describes the change within the time collection that happens over an extended time frame and is extra systematic. It displays the basic improve or lower within the collection and offers a sign of the general pattern in addition to the magnitude of the info collected over a protracted time interval. It’s useful in figuring out the underlying sample of the info in addition to the directionality of the info. In Determine 1, now we have the seasonality decomposition of income over 8 months, and if we take a look at the pattern we observe that there’s a decline in income throughout the summer season months of the yr. This perception could be essential for advertisers as they will devise a change in technique or their spending sample.
Seasonality Issue
The seasonality issue refers to recurrent patterns that happen over shorter durations of time, usually inside the span of 1 yr. It’s a illustration of the frequent oscillations that happen on account of exterior influences such because the climate, holidays, or different cultural occasions. The recurrent peaks and valleys which can be attribute of seasonality are a mirrored image of the regularities that may be anticipated inside the information. In Determine 1 above we are able to see that there are peaks each alternate month which may help information companies to determine some exterior influences having an impression on income.
Residual Issue
The random and unexplained variations that can not be attributed to the pattern or seasonality are represented by the residual element, which is often known as the error or noise element. It takes under consideration any fluctuations or anomalies which can be nonetheless current after the pattern and seasonality elements have been considered. The fraction of the info that’s unpredictable and lacks a scientific sample is denoted by the residual element.
Challenges confronted in seasonality evaluation
- A number of seasonalities: In sure time collection information one can observe a number of seasonality patterns at a day by day, weekly in addition to month-to-month stage which is troublesome to seize with a easy seasonal decomposition and should require extra complicated processes.
- Information sparsity: If we shouldn’t have evenly distributed information over a time frame i.e., if now we have sometimes sampled information or only a few information factors then it would impression the seasonality estimation. Therefore, it is suggested to have a day by day or weekly stage dataset for a minimum of 2 years for higher high quality seasonality estimation.
- Non-stationarity: In case the time collection information has a altering variance then will impression seasonality estimation.
Restricted or sparse information factors inside a specific season can hinder the correct estimation of seasonal results, particularly when coping with shorter time collection or sometimes sampled information.
- Irregularities: Usually instances now we have outliers in information as a consequence of some exterior components which may distort the seasonality evaluation. It’s suggested to carry out information screening previous to performing seasonality evaluation comparable to outlier detection and elimination.
Lastly, we noticed how seasonality influences media combine modeling and drives strategic enterprise choices. Entrepreneurs can optimize their promoting techniques and price range allocation primarily based on swings in client conduct and market dynamics all year long by together with seasonality in media combine fashions. Understanding seasonal patterns permits corporations to focus on the proper demographic, select the most effective media channels, and time their promoting campaigns for max impression. Firms could enhance the effectivity and efficacy of their promoting actions, improve buyer engagement, generate gross sales, and finally enhance their return on investments by using seasonality evaluation data. Seasonality allows corporations to regulate and tailor their advertising techniques to correspond with shifting client calls for and preferences, giving them a aggressive benefit in a unstable setting.
Mayukh Maitra is a Information Scientist at Walmart working within the media combine modeling area with greater than 5 years of business expertise. From constructing Markov course of primarily based outcomes analysis fashions for healthcare to performing genetic algorithm primarily based media combine modeling, I have been concerned in not solely making an impression within the lives of individuals but additionally taking companies to the subsequent stage by way of significant insights. Previous to becoming a member of Walmart, I’ve had the chance to work as a Information Science Supervisor in GroupM within the advert tech area, Senior Affiliate of Resolution Science in Axtria working within the area of well being economics and outcomes analysis, and as a Expertise Analyst in ZS Associates. Along with my skilled roles, I’ve been a part of jury and technical committee for a number of peer reviewed conferences, have had the chance to guage a number of tech awards and hackathons as nicely.