Within the evolving world of robotics, a groundbreaking collaboration between Princeton College and Google stands out. Engineers from these prestigious establishments have developed an modern technique that teaches robots an important talent: recognizing once they need assistance and the way to ask for it. This improvement marks a major leap ahead in robotics, bridging the hole between autonomous functioning and human-robot interplay.
The journey in direction of extra clever and impartial robots has at all times been hindered by one vital problem: the complexity and ambiguity of human language. In contrast to the binary readability of pc codes, human language is riddled with nuances and subtleties, making it a labyrinth for robots. As an illustration, a command so simple as “decide up the bowl” can turn into a fancy activity when a number of bowls are current. Robots, outfitted to sense their atmosphere and reply to language, usually discover themselves at a crossroads when confronted with such linguistic uncertainties.
Quantifying Uncertainty
Addressing this problem, the Princeton and Google staff has launched a novel strategy that quantifies the ‘fuzziness’ of human language. This system basically measures the extent of uncertainty in language instructions and makes use of this metric to information robotic actions. In conditions the place a command would possibly result in a number of interpretations, the robotic can now gauge the extent of uncertainty and determine when to hunt additional clarification. As an illustration, in an atmosphere with a number of bowls, the next diploma of uncertainty would immediate the robotic to ask which bowl to select up, thereby avoiding potential errors or inefficiencies.
This strategy not solely empowers robots with a greater understanding of language but additionally enhances their security and effectivity in activity execution. By integrating giant language fashions (LLMs) like these behind ChatGPT, the researchers have taken a major step in aligning robotic actions extra carefully with human expectations and desires.
Function of Massive Language Fashions
The combination of LLMs performs a pivotal position on this new strategy. LLMs are instrumental in processing and decoding human language. On this context, they’re used to guage and measure the uncertainty current in language instructions given to robots.
Nevertheless, the reliance on LLMs is not with out its challenges. As identified by the analysis staff, outputs from LLMs can generally be unreliable.
Anirudha Majumdar, an assistant professor at Princeton, emphasizes the significance of this steadiness:
“Blindly following plans generated by an LLM may trigger robots to behave in an unsafe or untrustworthy method, and so we want our LLM-based robots to know when they do not know.”
This highlights the need for a nuanced strategy, the place LLMs are used as instruments for steering moderately than infallible decision-makers.
Sensible Software and Testing
The practicality of this technique has been examined in numerous eventualities, illustrating its versatility and effectiveness. One such check concerned a robotic arm, tasked with sorting toy meals objects into totally different classes. This straightforward setup demonstrated the robotic’s capability to navigate duties with clear-cut decisions successfully.
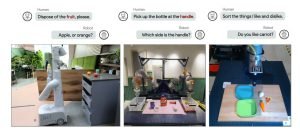
Picture: Princeton College
The complexity elevated considerably in one other experiment that includes a robotic arm mounted on a wheeled platform in an workplace kitchen. Right here, the robotic confronted real-world challenges like figuring out the right merchandise to position in a microwave when offered with a number of choices.
By means of these assessments, the robots efficiently demonstrated their capability to make use of the quantified uncertainty to make choices or search clarification, thereby validating the sensible utility of this technique.
Future Implications and Analysis
Wanting forward, the implications of this analysis prolong far past the present purposes. The staff, led by Majumdar and graduate scholar Allen Ren, is exploring how this strategy will be utilized to extra advanced issues in robotic notion and AI. This contains eventualities the place robots want to mix imaginative and prescient and language data to make choices, additional closing the hole between robotic understanding and human interplay.
The continued analysis goals to not solely improve the power of robots to carry out duties with larger accuracy but additionally to navigate the world with an understanding akin to human cognition. This analysis may pave the best way for robots that aren’t solely extra environment friendly and safer but additionally extra in tune with the nuanced calls for of human environments.
You’ll find the revealed analysis right here.