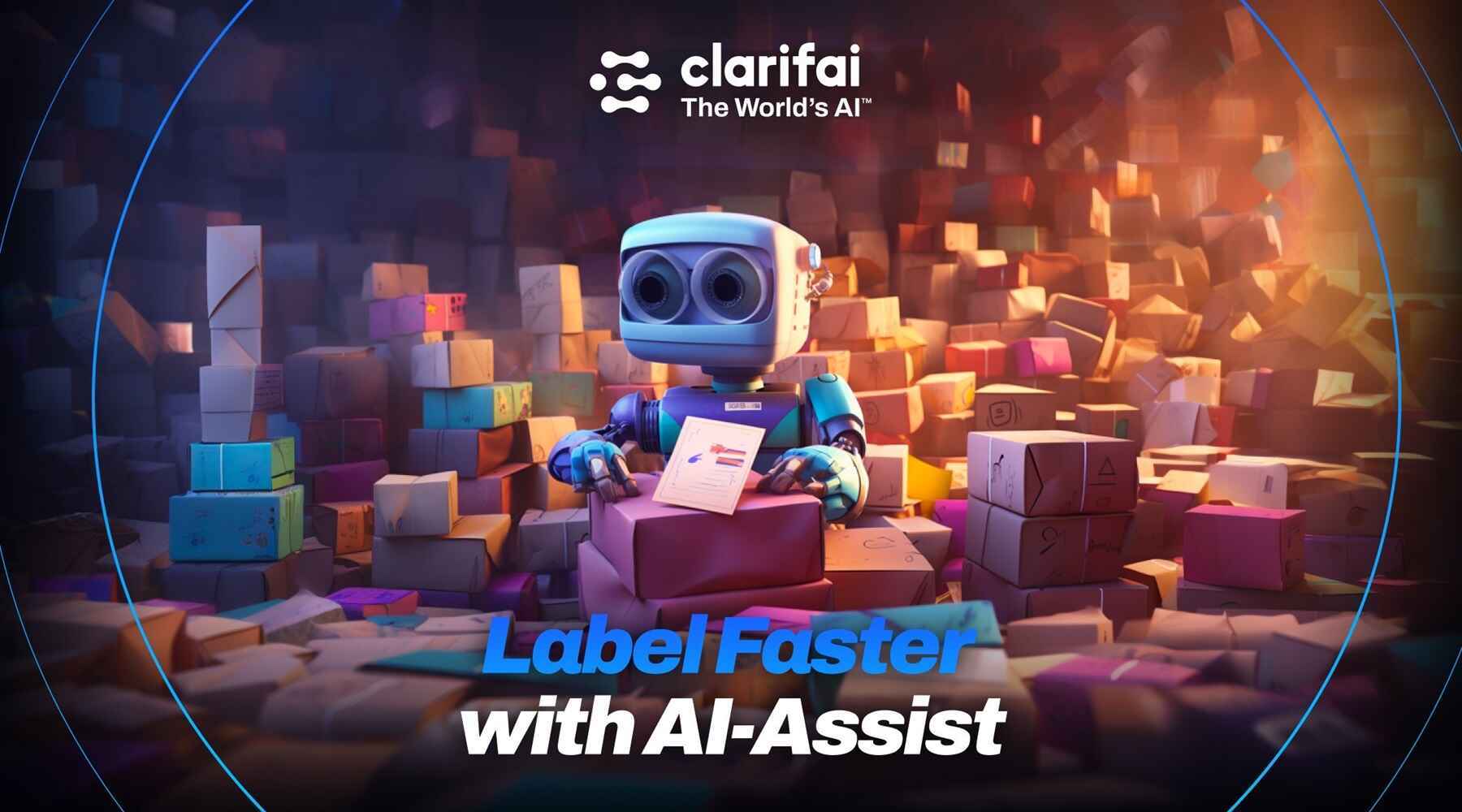
The Rise of AI-Assisted Labeling
Knowledge labeling is an indispensable aspect within the machine-learning ecosystem. For fashions to be educated successfully, they require clear, labeled information. Through the years, information labeling has undergone a big transformation, mirroring the speedy developments in expertise and our understanding of machine studying methodologies.
Within the early days of machine studying, information labeling was predominantly guide. Researchers and builders would sit for hours, meticulously assigning labels to particular person information factors. For example, in picture recognition duties, photos could be manually tagged with their respective classes, be it “cat,” “canine,” “automotive,” and so forth. This course of was time-consuming and susceptible to human errors and inconsistencies.
As datasets grew bigger, guide labeling turned much more difficult. This led to crowd-sourced platforms, like Amazon Mechanical Turk, the place many customers may contribute to labeling duties. Whereas this decentralized strategy addressed the amount problem considerably, it launched one other layer of complexity in sustaining consistency and high quality.
The concept of AI-assisted labeling started to take root within the late 2010s as machine-learning fashions turned extra refined. Researchers realized that as a substitute of ranging from scratch with each new batch of information, beforehand educated fashions might be leveraged to make preliminary predictions or “solutions” for labels. This did not get rid of the necessity for human intervention however considerably diminished the guide labor concerned. The human labelers may then validate and proper these solutions, making certain accuracy. This hybrid strategy was each environment friendly and efficient.
The inception of AI-assisted labeling was a game-changer, particularly for large-scale tasks. Mixed with the computational prowess of algorithms and the discerning judgment of human specialists, it was now attainable to label huge datasets extra precisely and in a fraction of the time beforehand required.
Clarifai has embraced and enhanced this method, integrating AI-assisted labeling into our toolsets, making the labeling course of much more streamlined and intuitive for builders and AI fanatics. Given the hyperlink between information high quality and the efficiency of ML fashions, it’s crucial for AI builders to prioritize top-notch information annotation options proper from the beginning of their tasks.
At Clarifai, we’re continuously releasing new options that assist AI builders optimize their coaching information manufacturing throughput, effectivity, and high quality. Let’s check out how our newly launched AI-Help options can assist you spend much less time labeling and extra time coaching.
What’s Mannequin-Assisted Labeling?
Mannequin-assisted labeling (MAL) is a way wherein predictions from machine studying fashions are used to help or increase the human labeling course of. It’s typically employed throughout the context of broader human-in-the-loop (HITL) mannequin improvement workflows, which may be understood as an iterative, human-machine course of sometimes involving the next steps:
- People add ground-truth annotations to unlabeled information
- ML fashions are educated in a supervised vogue on labeled information
- Predictions from educated fashions are used to annotate new unlabeled information upon human verification
The HITL course of operates as a steady suggestions loop, the place the coaching, tuning, and testing duties repeatedly inform and enhance the algorithm. This iterative strategy enhances the algorithm’s effectiveness and accuracy over time, leading to extremely exact and in depth coaching information for particular use circumstances. The human involvement on this course of is instrumental. Organizations can obtain probably the most correct and actionable decision-making capabilities by fine-tuning and testing their fashions iteratively.
Benefits of HITL
Past the benefits of precision and accuracy, the HITL methodology additionally introduces important financial efficiencies into the machine studying lifecycle. Integrating people and AI in an iterative cycle presents a mix of human experience and computational effectivity, which has far-reaching value implications.
Firstly, there’s the matter of manpower prices. Conventional information labeling and mannequin refining processes with out AI help typically require in depth human sources. With the HITL strategy, AI assists within the preliminary labeling levels, decreasing the reliance on massive annotating groups. Consequently, tasks can obtain substantial financial savings, particularly when coping with huge datasets that will in any other case demand important man-hours.
Time-related financial savings are one other dimension value highlighting. In conventional setups, the prolonged durations of labeling and refining can push again mannequin deployment, inflicting a ripple impact of delayed ROI realization. Nevertheless, the HITL course of, with AI’s acceleration, ensures faster label validations and mannequin refinements. Because of this, tasks not solely keep their high quality requirements but additionally adhere to tighter timelines, enabling companies to deploy their AI options extra promptly. This accelerated deployment is pivotal for organizations in aggressive markets, the place the first-mover benefit can dictate market share and profitability.
Infrastructural overhead historically related to large-scale information processing tasks can also be considerably diminished. With the HITL mannequin’s streamlined strategy, there’s much less of a necessity for expansive infrastructure. Clarifai’s platform presents the added benefit of scalability with out the accompanying capital expenditure, decreasing the direct prices related to infrastructure and slicing down on overheads like upkeep, administration, and utilities.
Placing AI-Help to work
In contrast with current Good Search and Bulk Labeling options that can be utilized throughout the Enter-Supervisor display to speed up labeling, our latest AI-Help options may be employed whereas working with particular person Inputs throughout the Enter-Viewer display.
To start out, merely choose an current Mannequin or Workflow (both owned by you or from Clarifai’s Neighborhood) throughout the Annotate Mode settings within the right-hand sidebar.
After selecting a mannequin or workflow, advised Annotations are sorted in descending order based mostly on confidence rating.
From right here, solutions may be accepted individually or in bulk utilizing the minimal confidence threshold slider.
Choosing the Finest Annotation Software For Your Job
Selecting the best labeling instruments on your AI improvement group is a vital resolution that may considerably affect your mission’s success. You possibly can both develop your personal customized device set or leverage current business instruments. Nevertheless, constructing in-house instruments may be advanced and time-consuming, particularly if you happen to hope to make the most of them in human-in-the-loop mannequin improvement workflows.
In comparison with in-house tooling, Clarifai’s labeling instruments make it simpler to leap into labeling your information at a fraction of the upfront value. Our labeling instruments are repeatedly evolving, with new options like AI-Help designed to reinforce label high quality whereas minimizing the hassle required out of your group. That’s why we’re constructing an end-to-end AI improvement platform that ensures you get the perfect outcomes attainable out of your ML fashions. For those who’re seeking to practice and deploy high-quality ML fashions in probably the most environment friendly approach attainable, join a free Clarifai Neighborhood account at the moment!